1. INTRODUCTION
Significant advances in the field of assisted reproductive technology (ART) have helped numerous infertile couples fulfil their desire for parenthood. In its most recent report, the International Committee Monitoring Assisted Reproductive Technologies (ICMART) estimated that around 1.9-2.2 million ART cycles were being performed globally each year, with 480,000 babies delivered annually [1]. Between the birth of the first test-tube baby, Louise Brown, in 1978 and 2012, the total number of babies born via ART has reached 6.5 million worldwide. Despite these impressive numbers, not every couple who present for fertility treatment will be successful. On average, only half of all women starting IVF will achieve a successful live birth, with the rest remaining childless despite multiple IVF cycles [2].
In view of the considerable physical, emotional and financial burden associated with IVF, it is important to discuss the probability of success with each couple prior to their decision to commit to IVF. Common prognostic factors known to influence the live birth rate include the woman’s age, cause and duration of infertility, ovarian reserve markers such as antral follicle count (AFC), anti-Mullerian hormone (AMH) levels, the number of oocytes retrieved, and embryo- related factors [3-5]. To aid physicians and patients in their decision-making process, one or more of these prognostic factors can be statistically modelled to predict IVF outcome. The power of prediction increases when several prognostic factors are used together [6, 7].
Most existing models were developed to predict pregnancy or ongoing pregnancy as the primary outcome [8, 9]. Very few have attempted to predict live birth following IVF [10-13] and only two, the Templeton [13] and Nelson [11] models, have been externally validated. However, both these models are of limited use in Asian countries because their datasets were derived from populations predominantly consisting of Caucasian women. Current evidence suggests that Asian women experience significantly lower clinical pregnancy and live birth rates with IVF compared with Caucasian women, probably due to physiologic and socioeconomic differences [14, 15]. In addition, the Templeton model pre-dated the use of ICSI whilst the model by Nelson involved fresh cycles only.
With the gradual shift from use of long gonadotropin- releasing hormone (GnRH) agonist protocols to a GnRH antagonist protocol as the standard regimen [16], plus the widespread use of ICSI and embryo vitrification in modern ART treatment [17], there is a need for newer and more accurate models to facilitate patient selection, guide clinical decisions and improve patient counselling. In this study, we aimed to develop a reliable prediction model to estimate the probability of a live birth at 12 months in patients who had completed one IVF/ICSI cycle (including all fresh and frozen embryo transfers from the same oocyte retrieval) in a controlled ovarian cycle using GnRH antagonist stimulation protocol.
2. MATERIALS AND METHODS
In this retrospective cohort study, we retrieved data for women who had undergone ART treatment at IVFMD, My Duc General Hospital, Ho Chi Minh City, Vietnam, between April 2014 and December 2015. Women included in the development cohort fulfilled the following criteria: age 18-45 years, embryo transfer(s) within 12 months since the start of the IVF cycle, and a maximum of 2 embryos for transfer on Day 3. Women who had IVF with donor eggs, in-vitro maturation of oocytes, cancelled cycle due to poor ovarian response or no oocyte pickup (OPU), no embryo available for transfer and uterine abnormalities (i.e. adhesion of the uterine cavity, bicornuate uterus or didelphis uterus) were excluded.
All subjects received recombinant follicle-stimulating hormone (rFSH) stimulation in a GnRH antagonist protocol. rFSH was administered from Day 2 of the menstrual cycle at an initial dose of 150 IU, 225 IU or 300 IU per day based on the patient characteristics, including age, body mass index (BMI), AMH level and AFC. Stimulation was continued until the day of human chorionic gonadotrophin (hCG) administration; daily rFSH dose was titrated at the discretion of the attending physician. Ovarian response was monitored by serial ultrasound scan and hormone levels (serum estradiol and progesterone). hCG (250 μg/0.5 mL, Ovitrelle®; Merck Serono, Germany) was administered when at least 2 leading follicles had reached a diameter of 17 mm on ultrasound.
GnRH agonist (triptorelin 0.2 mg subcutaneous injection, Decapeptyl®; Ipsen Beaufour, France) was used for triggering oocyte maturation when there were more than 15 developing follicles on the day of trigger. Oocyte retrieval was performed via transvaginal aspiration 36 hours after triggering.
ICSI was performed for all cycles. Fertilization was checked 16-18 hours post-insemination. Embryos were rated according to the Istanbul Consensus criteria, where “good” was defined as having Grade 1 morphology (i.e. presence of 8 cells, even cell size, less than 10% fragmentation and no multinucleation) [18]. Embryo transfer occurred on Day 3 after oocyte retrieval. The choice of single or double fresh embryo transfer was based on the number of good-quality embryos available. Additional embryos were cryopreserved for later use. Indications for a freeze-all strategy were GnRH agonist trigger, unfavorable endometrium, fluid accumulation in the endometrial cavity, hydrosalpinx not removed before IVF treatment, risk of ovarian hyperstimulation or patient preference. Patients who failed the first fresh or frozen transfer were given the option to return for subsequent FET.
Patients who had hCG trigger cycle were given daily vaginal pessary progesterone 400 mg (Cyclogest®; Actavis, UK) for 16 days starting from the day of OPU. For patients who underwent GnRH agonist trigger, luteal support consisted of a combination of intramuscular progesterone 50 mg per day (Rotex Medica, Germany), vaginal suppository progesterone 400 mg per day and oral estradiol valerate 6 mg per day (Progynova®; Bayer Schering, Germany) from the day of OPU. Pregnancy testing was performed 14 days after embryo transfer. A serum beta-hCG of more than 5 mIU/mL was considered positive for pregnancy. Clinical pregnancy was confirmed via transvaginal ultrasonography at 7 weeks of gestation.
The primary outcome for the model was live birth, defined as the birth of a newborn after 24 weeks’ gestation that exhibited any sign of life, such as respiration, heartbeat, umbilical pulsation or movement of voluntary muscles. The birth of twins was considered as a single live birth event.
We assessed the predictive ability of the three models based on two performance measures, i.e. discrimination and calibration. The ability to distinguish patients who will achieve live birth from those without live birth at 12 months after starting IVF was tested using receiver operating characteristics (ROC) analysis. For calibration, we compared the predictive values of the model with real observed live birth rates. To provide clinicians with a user-friendly interface for the final model, we constructed a nomogram that could be used as a graphical tool for conveying the probability of birth based on an individual patient profile.
Data were analysed using the R Statistical Environment version 3.3.3 on Windows platform. Firstly, descriptive analysis was applied to the development cohort, and then a bi-variable followed by multi-variable Cox proportional hazards regression model was used to determine factors that may affect the probability live birth within 12 months of starting IVF treatment. We used the following routinely recorded variables for testing:
-
■ Pre-treatment factors: female age, BMI, baseline AMH and AFC, number of prior IVF attempts, IVF indications, duration of infertility and type of infertility.
-
■ Treatment factors: duration of stimulation, total dose of rFSH, type of trigger (hCG or GnRH agonist), estradiol level on day of trigger, progesterone level on day of trigger, and the number of oocytes retrieved.
-
■ Laboratory factors: total number of embryos obtained, number of good-quality embryos.
-
■ Factors associated with embryo transfer: mode of transfer (fresh or frozen transfer during the first transfer), number of subsequent FET after first transfer.
Variables with a p value of ≤0.25 were selected for multi-variable analysis. To reduce the number of variables for the final model to predict live birth, we conducted Pearson’s correlation analysis for paired samples. A coefficient of correlation of 0.7 (P < 0.05) was obtained for the following data pairs:
-
■ Number of oocytes retrieved and total number of embryos obtained
-
■ Total number of embryos obtained and number of good- quality embryos
-
■ Number of embryos and number of subsequent FET after the first transfer
-
■ Number of good-quality embryos and number of subsequent FET after the first transfer
Only one variable from each data pair was selected. Because the number of oocytes retrieved led to the embryology outcomes, and the number of subsequent FET after the first transfer directly correlates with the probability of live birth, these two variables were included for multi- variable Cox regression. Therefore, there were a total of 14 variables for regression modelling. From these 14 variables, we can expect a total of 214 = 16,384 models to be developed.
The study was approved by the Institutional Review Board (approval number CS/MD/17/13). The IVFMD database contained information that was routinely collected from all patients, including baseline demographics, cycle data and outcome data. Patient information was kept confidential and patient consent was obtained at the start of IVF treatment allowing use of their data for research purposes. No medical ethical approval was required for this research.
3. RESULTS
FromApril 2014 to December 2015, a total of 4551 women underwent IVF/ICSI at My Duc Hospital. Approximately 2810 women (61.7%) fulfilled the inclusion/exclusion criteria and formed the development cohort. Two hundred and ten women returned to their home town to give birth and could not be contacted and were therefore lost to follow- up, leaving a dataset of 2600 women for model development (Figure 1). Table 1 shows the baseline demographics and IVF cycle characteristics.
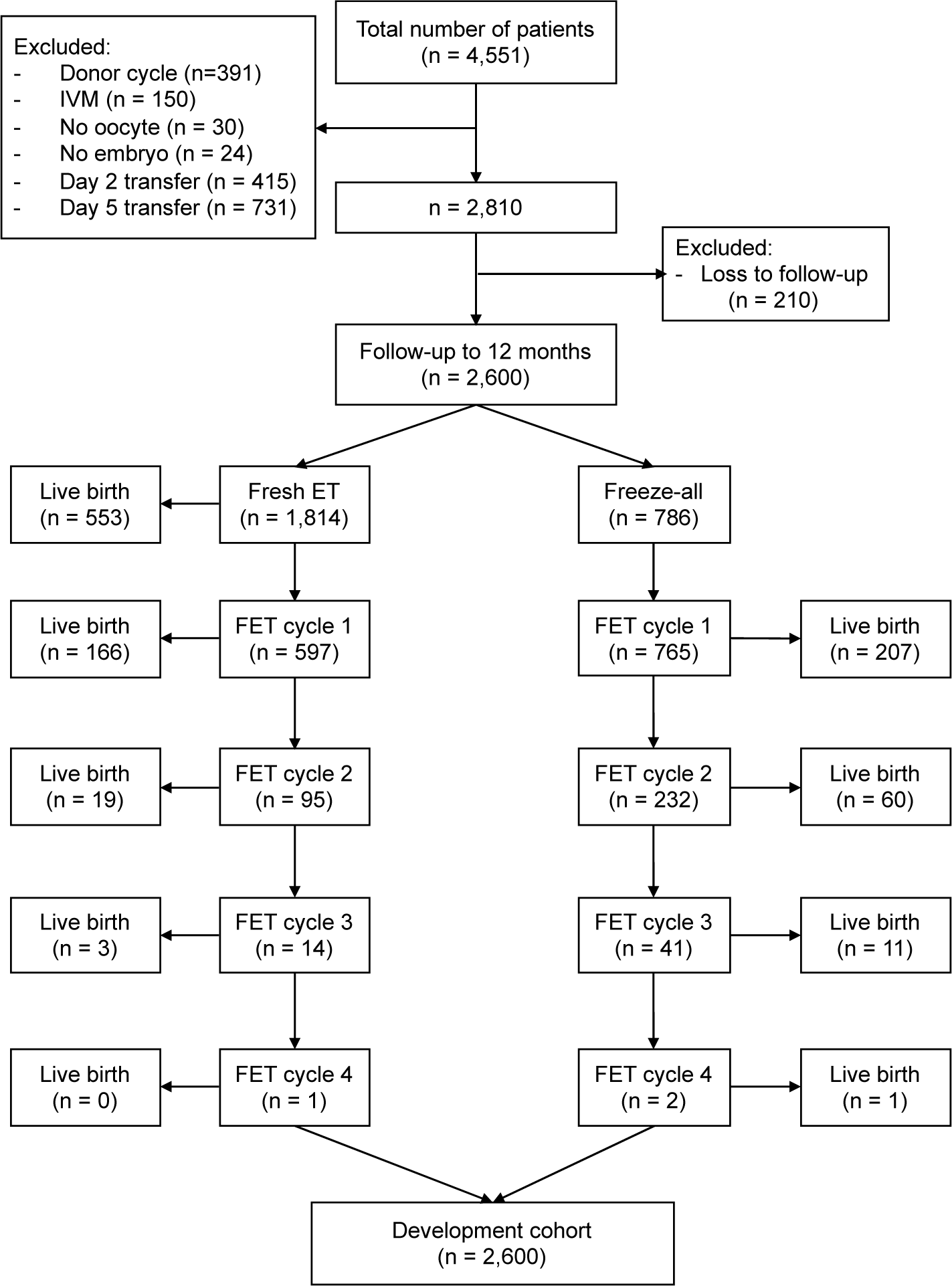
The overall rate of at least one live birth from the whole dataset was 39.2%. Live birth rate per cycle (inclusive of all fresh and frozen embryo transfers) at 12 months was 28.6%. Life birth rate was 50.0% for women aged ≤25 years, 45.5% for women aged 26-30 years, 40.2% for women aged 31-35 years, 30.7% for women aged 36-40 years and 8.2% for women aged >40 years. The cumulative live birth rate in women who had undergone fresh embryo transfer followed by subsequent FET cycle(s) was 40.8%. This was higher than the 35.5% live birth rate for patients managed using an initial freeze-only strategy.
Bi-variable associations between potential predictors for live birth at 12 months following IVF are shown in Table 2. There were sixteen important predictive variables: female age, AMH, AFC, duration of infertility, type of infertility, IVF indications, number of IVF attempts, duration of stimulation, total dose of rFSH used, type of trigger, progesterone level on the day of trigger, number of oocytes retrieved, number of embryos, number of good embryos, fresh or frozen transfer for the first transfer, and the number of subsequent FET after the first transfer. We applied Bayesian model averaging to all possible models (Figure 2), using the Bayesian informative criteria (BIC) approximation and posterior probability for ‘best model’ selection. A model that has the lowest BIC and highest posterior probability is considered the best. Therefore, we selected the 3 best models, which had comparable BIC and posterior probability (Table 3); the remaining two models had higher BIC and lower posterior probability:
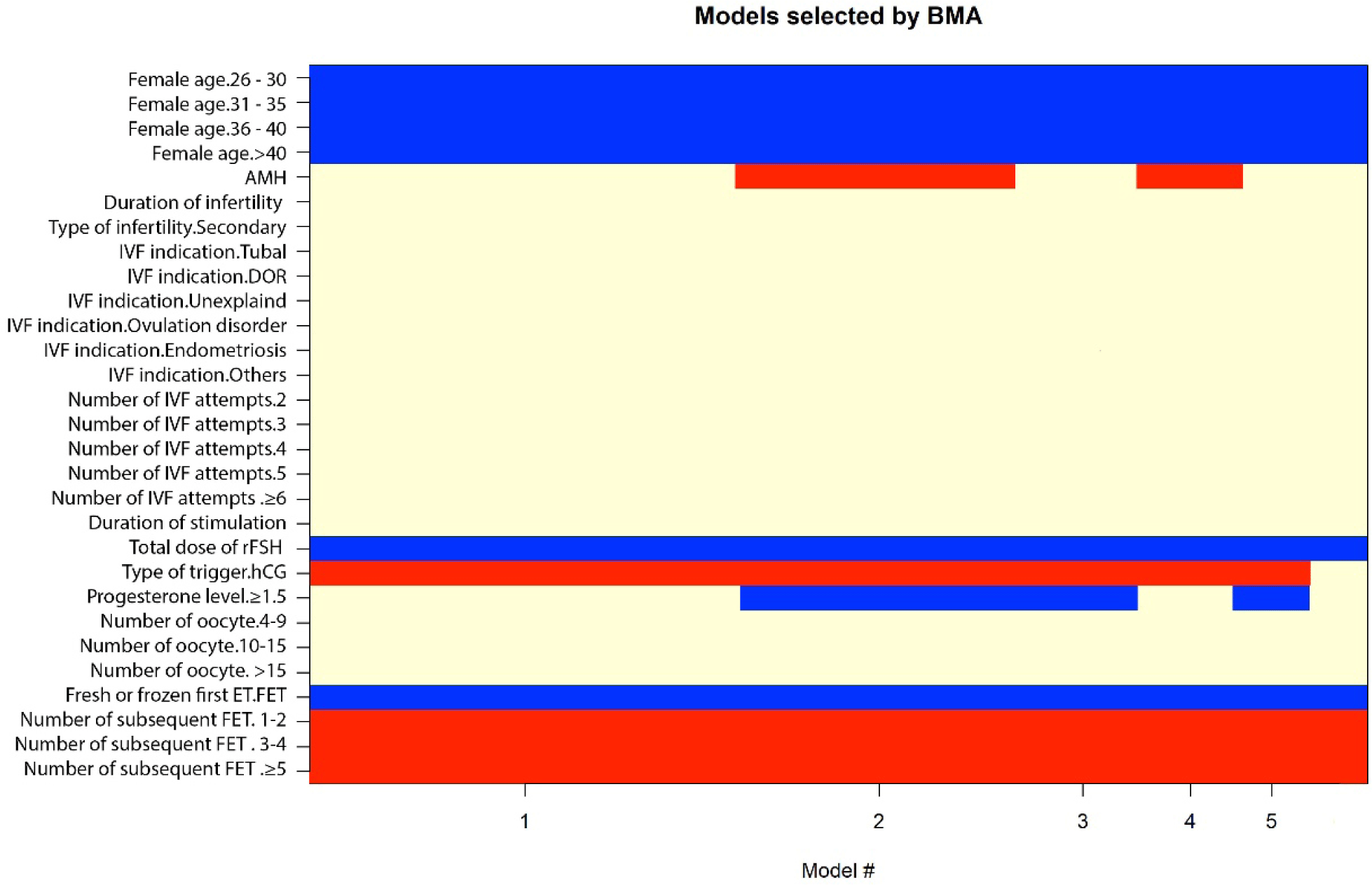
-
Model I consisted of five predictive factors: female age, total dose of rFSH used, type of trigger, fresh or frozen transfer during the first transfer, and number of subsequent FET after the first transfer.
-
Model II consisted of seven predictive factors: female age, AMH, total dose of rFSH used, type of trigger, fresh or frozen transfer during the first transfer, number of subsequent FET after the first transfer, and progesterone level on the day of trigger.
-
Model III consisted of six predictive factors: female age, total dose of rFSH used, type of trigger, fresh or frozen transfer during the first transfer, number of FET after the first transfer, and progesterone level on the trigger day.
Missing data occurred for BMI (1.03%), AMH (3.42%), AFC (9.42%), duration of infertility (6.73%), and estradiol and progesterone levels on the day of trigger (6.31% and 6.54%, respectively) (Figure 3). To avoid potential bias and statistical inaccuracy due to loss of data, we used multi-variable imputation by chained equation (MICE) method to complete multi-variable predictors with missing data. The MICE algorithm generates plausible synthetic values for predictors with missing information within a column, conditional on all other columns in the data. For this purpose, we assumed that the missing data occurred randomly. Imputation was performed using R package mice (version 2.30)
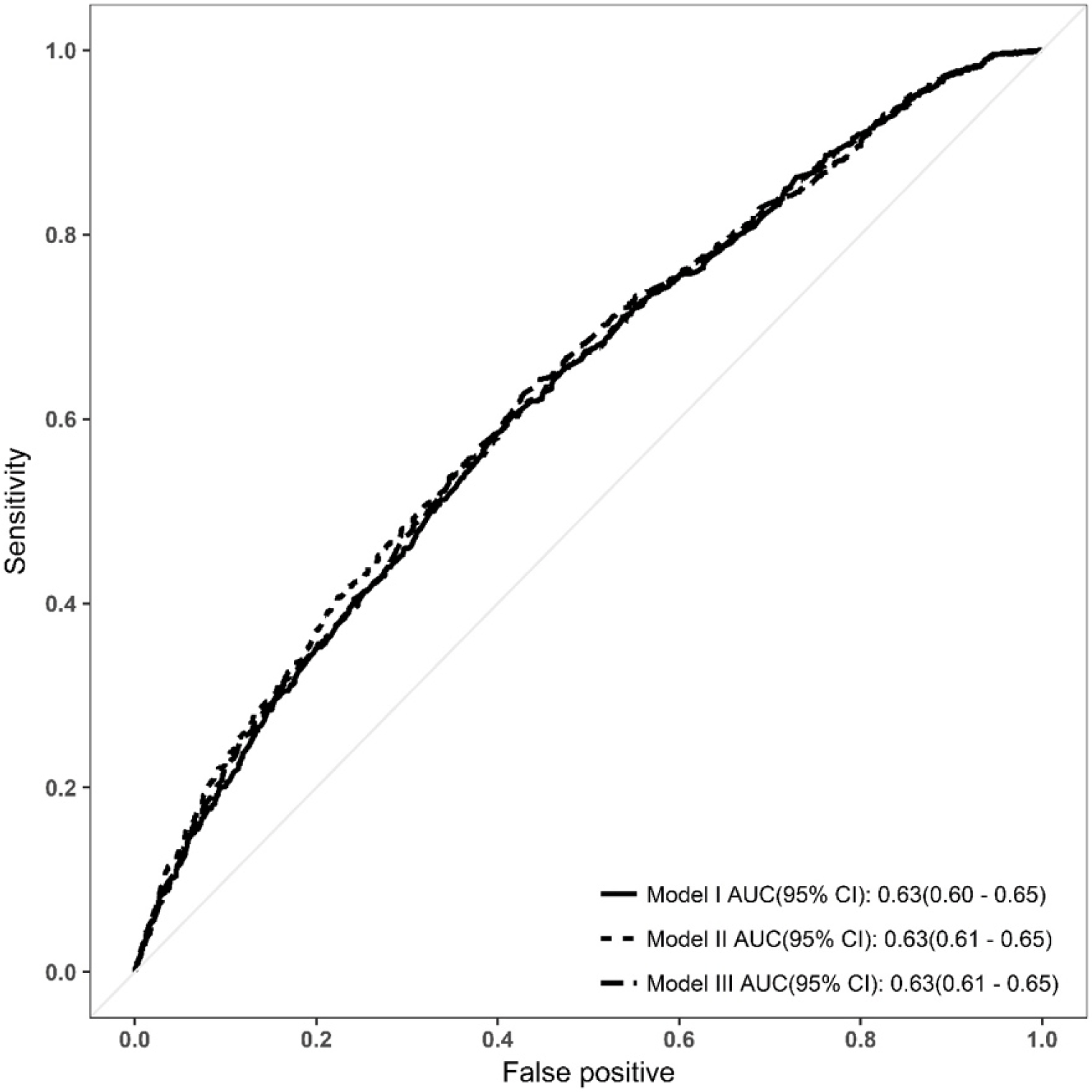
There were no significant differences in the area under the ROC curve (AUROC) between the three models (DeLong test, p > 0.05) (Figure 3). Discrimination, assessed by determining the AUC, was similar for all three models. The AUC was 0.63 (95% confidence interval [CI] 0.60-0.65) for Model I, and 0.63 (95% CI 0.61–0.65) for Models II and III. The calibration plots for all three models showed good agreement between the predicted and observed probabilities of live birth (Hosmer-Lemeshow test, p > 0.05) (Figure 4).
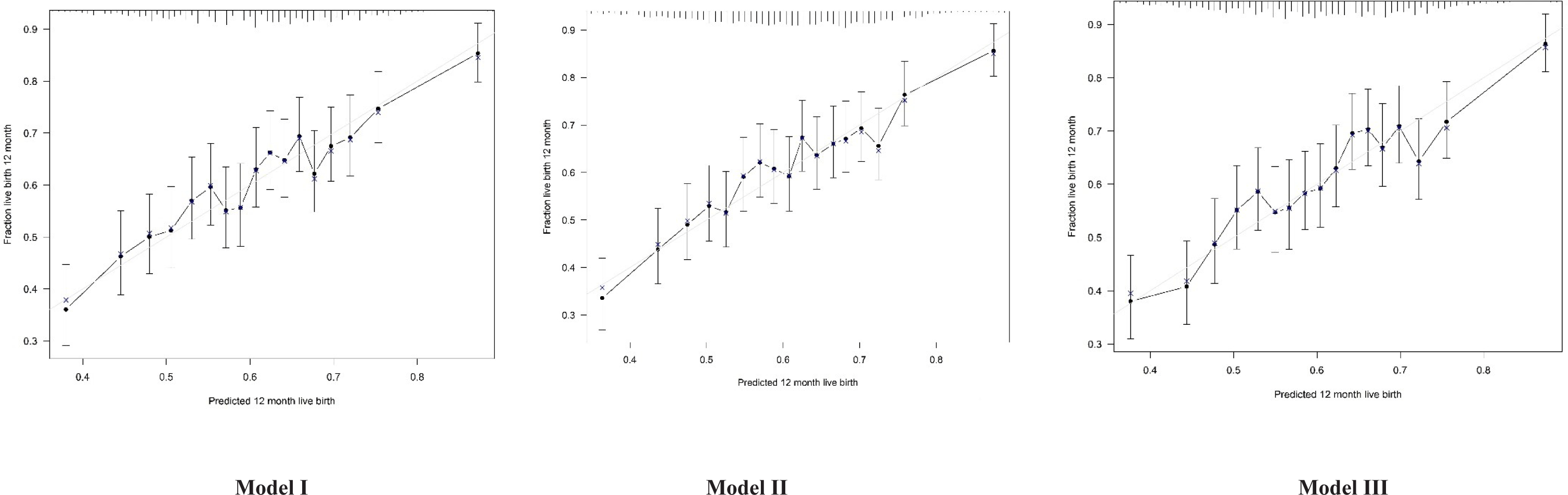
To validate Models I, II and III, we obtained data from a new cohort of patients undergoing IVF treatment at the same centre over different period (January to June 2016). A total of 1416 patients met the inclusion and exclusion criteria (Figure 5). The baseline demographics and IVF cycle characteristics of the validation cohort were comparable to those of the development cohort (Table 1). The overall birth rate of the validation cohort was 38.3% (543/1416), which was comparable to the live birth rate of the development cohort (39.2%). When Models I, II and III were applied to the validation cohort, the AUC was 0.60 (95% CI 0.57-0.63) for all three models (Figure 6). This was slightly lower than the value obtained from the development cohort.
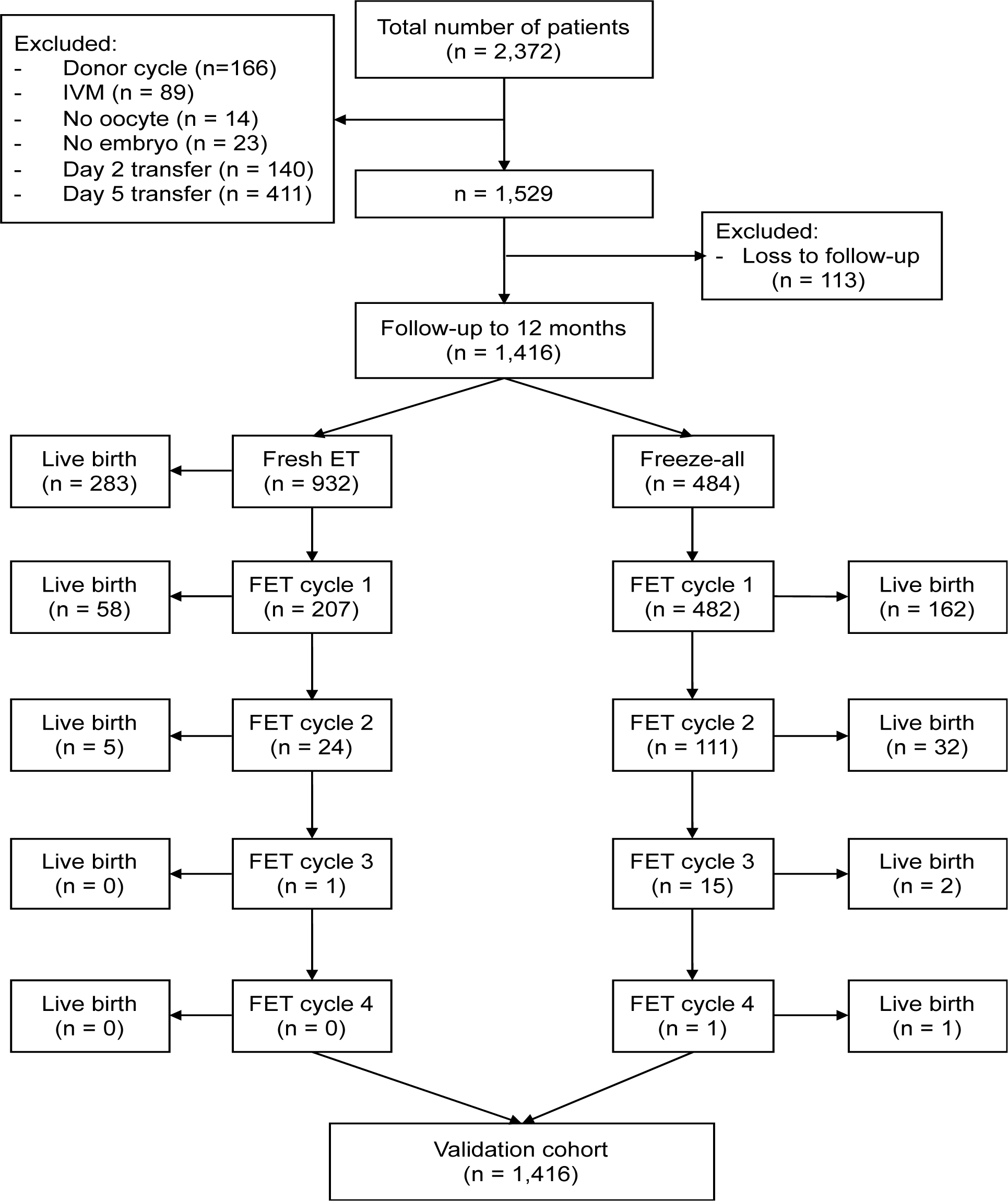
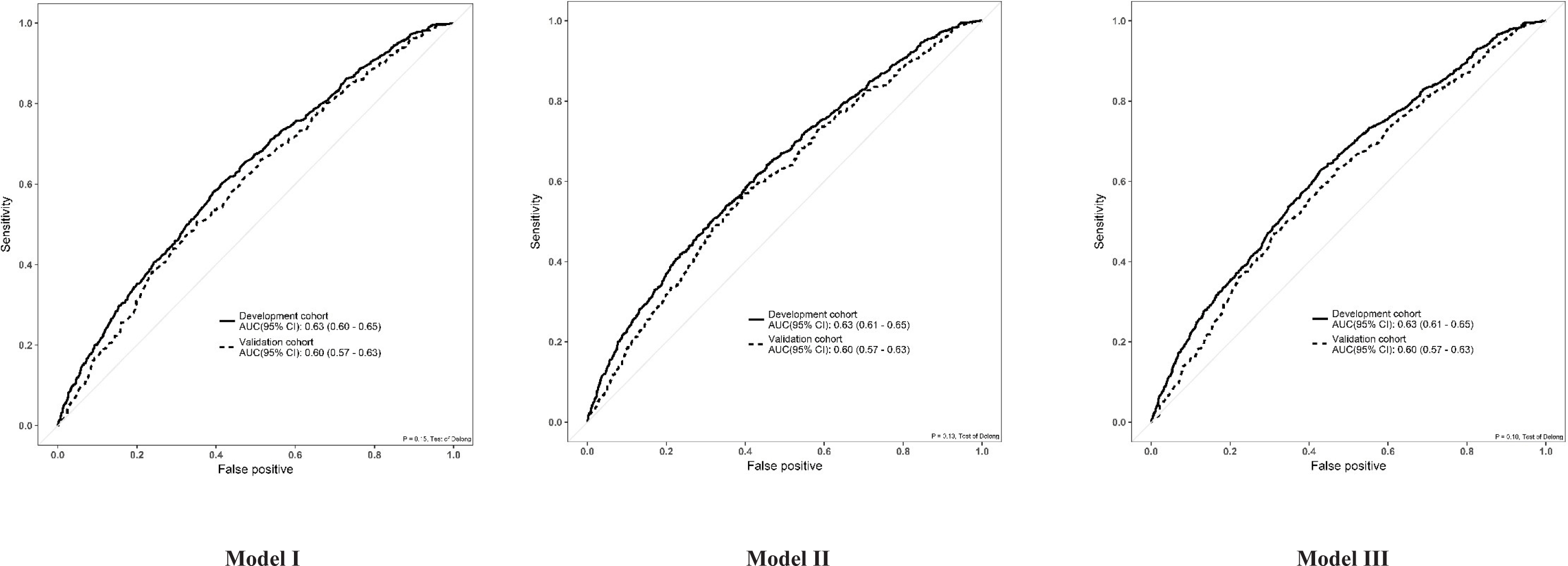
All three models were comparable with regards to BIC, posterior probability, performance and validation. We selected Model I as the most parsimonious model because it required the least number of predictive factors for input. The nomogram showing probability of live birth in an individual patient is shown in Figure 7. The C-index for the nomogram is the AUCROC of Model I (0.63, 0.60–0.65).
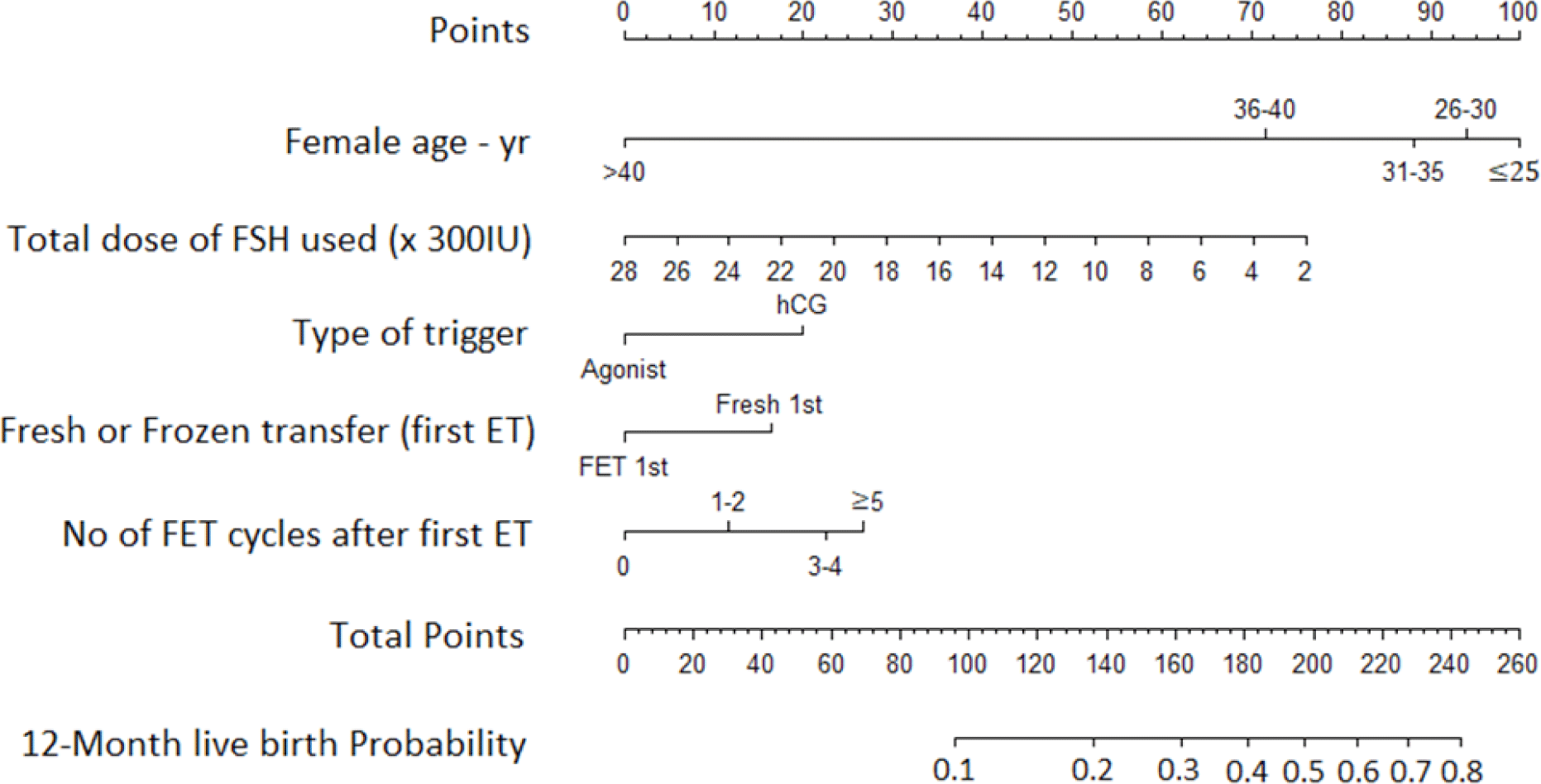
4. DISCUSSION
In our study, we identified five factors that significantly influenced th e probability of live birth. As expected, age was one of the most important factors influencing the outcome of IVF/ICSI. The hazard ratio for live birth in women over the age of 40 years was considerably lower at 0.15 (95% CI 0.07-0.31, p < 0.001) versus 0.59 (95% CI 0.44-0.78, p < 0.001) in the 36-40 years age group. In the bi-variable analysis, we demonstrated that baseline AMH and AFC were significantly associated with live birth. Studies have shown both AMH and AFC to be good predictors of ovarian response independent of age, suggesting that there may be a positive role for the inclusion of biomarkers for ovarian reserve in the prediction for live birth [19-21]. However, AMH and AFC were excluded from our final multi-variable model because we did not find any significant difference in discrimination when these variables were added either alone or together.
This was in agreement with the findings of another study [22], in which the combination of AMH (with or without AFC) and age only correctly classified an additional < 2% of subjects compared with the model including age alone.
Another important predictive factor identified in our study was the duration of infertility. The mean duration of infertility for women with no live birth was 5.0 ± 3.5 years compared with 4.6 ± 3.3 years for women with live birth. Women with a longer duration of infertility were less likely to achieve live birth, with a hazard ratio (HR) of 1.20 (95% CI 1.05-1.36, p = 0.007) for those who were infertile for ≥5 years versus those who were infertile for 4 years or less. We excluded this variable from the final model for the same reason as AMH and AFC. Another significant predictive factor was rFSH consumption. The mean total rFSH dose used was 2648.3 ± 909.9 IU for women with no live birth and 2424.4 ± 843.5 IU for those with live birth. For every increase of 300 IU rFSH, the probability of live birth was reduced by 37% (HR 0.93 [CI 95% 0.91-0.95], p < 0.001).
Not all women in our study used all their cryopreserved embryos within the 1-year follow-up period. Three hundred and eighteen women had not achieved live birth but still had remaining frozen embryos. In two different studies [23, 24], the minimum follow-up time from the start of IVF cycle was 2 years. Nevertheless, our follow-up period for assessing live birth at 12 months can be justified because preliminary analysis showed that the median time from the start of treatment to live birth at our center was 9.5 months. In addition, the proportion of patients returning after 12 months was low (<8%). Furthermore, IVF is not subsidized or covered by health insurance in Vietnam meaning that patients pay for their own IVF treatment and usually do not have the financial means to pursue treatment for up to 2 years.
To our knowledge, this is the first predictive model for live birth to be derived from an Asian population. We assessed the probability of live birth in women receiving ICSI and GnRH antagonist treatment, both of which are favoured and practiced in many countries of this region [25-29]. Existing multi-variable models for predicting live birth had been derived based on GnRH agonist cycles [12, 30], excluded ICSI or FET [11, 13], or were limited to fresh single embryo transfer when GnRH antagonist treatment was used [31, 32]. Hence, a major strength of our model is its relevance to current ART practices, particularly in the region from which data were used to generate the model. The nomogram developed is the first of its kind to allow prediction of live birth based on pre-treatment, post-stimulation, laboratory and embryo transfer parameters.
Of existing models that used live birth as the primary outcome, only the Nelson model has been validated in an Asian country (Singapore) [33]. However, this showed that the Nelson model fitted poorly with the local study population. This is likely due to differences in ethnicity and the legally-mandated younger age limit for IVF treatment (≤ 45 years). Unlike the Nelson model, ours was derived from an Asian population and included a younger age group (the maximum age for IVF treatment in our study was 45 years versus 50 years in the Nelson model). Therefore, our prediction model may have greater reproducibility and generalizability in countries whose population share similar age-related biological or physiological attributes with our patients.
We acknowledge several shortcomings in our study. Firstly, the number of women returning to use frozen embryos within 12 months after the first embryo transfer was low. In addition, the AUC of our final model and validation cohort demonstrated poor discriminative ability to separate women with and without live birth at 1 year with an AUC of 0.63 and 0.60, respectively. An AUC of 1 indicates perfect discrimination whereas an AUC of 0.5 indicates no discrimination. A model is considered to have poor performance if the AUC lies between 0.5 and 0.7, fair performance if the AUC lies between 0.7 and 0.8, and good performance if the AUC lies between 0.8 and 0.9 [9]. Existing models for live birth following IVF treatment that have been externally validated had shown similarly poor discrimination. The Templeton and Nelson models each had an AUC of 0.63 [11, 34], while the model by Dhillon et al. had an AUC of 0.62 [10]. For IVF models, achieving fair or good discrimination is unfeasible because the AUC typically does not exceed 0.67. As such, calibration is considered a more meaningful measure of model performance than discrimination [35]. Our model had good calibration as measured using the Hosmer-Lemeshow goodness-of-fit statistic, indicating close agreement between predicted and observed live births.
Another potential limitation is that the live birth rate may be affected by confounding factors that potentially arise as the pregnancy progresses. For example, prematurity and pregnancy complications, such as hypertension, gestational diabetes, and intrauterine growth retardation could reduce the probability of live birth. Lastly, as with any retrospective study designed to analyse pre-existing data, the presence of biases cannot be excluded [36]. To mitigate random error and statistical bias, we used a relatively large sample size (n = 2600) to construct this model.
It is important for clinicians to manage patient expectations about their chances for successful outcomes at different stages of the IVF cycle. The main function of our model is to create a patient counselling tool that accounts for all relevant predictors of live birth starting from the time of presentation to the day of embryo transfer. By collectively assessing these factors, clinicians will be able to provide patients with a more accurate prognosis than with pre- treatment information alone.
In places where access to IVF treatment is regulated by insurance reimbursement or legislative policies, patients with poor pre-treatment factors (e.g. advanced age or low ovarian reserve) are often denied IVF treatment due to their low chance of achieving live birth and advised to consider donor IVF or adoption instead. This is often contrary to the patients’ desire to have their own genetic offspring. From the clinician’s perspective, predicting reproductive outcomes is a dynamic process. For example, 35/2600 patients (1.3%) in our development cohort were aged >38 years and had AMH ≤1.25 ng/mL, but 22/35 (62.9%) had at least 1 good embryo for transfer. Therefore, the probability of live birth should be adjusted according to the patient’s response to IVF treatment up to the day of embryo transfer.
5. CONCLUSIONS
The discriminative ability of our model is comparable with previous models and shows good calibration. It has potential for implementation in clinical practice, particularly in settings where similar IVF/ICSI practices described in this study are employed. Our model should provide a more individualized and objective means for counselling patients about treatment outcomes following ovarian stimulation and oocyte retrieval, and to advise on the probability of live birth with fresh versus freeze-all cycles, as well as manage the patient’s expectations about subsequent FET cycles if the initial fresh transfer was unsuccessful. Future studies should include geographical external validation and impact analyses to evaluate the true benefits of integrating this model as part of the IVF patient care process.